Highlights
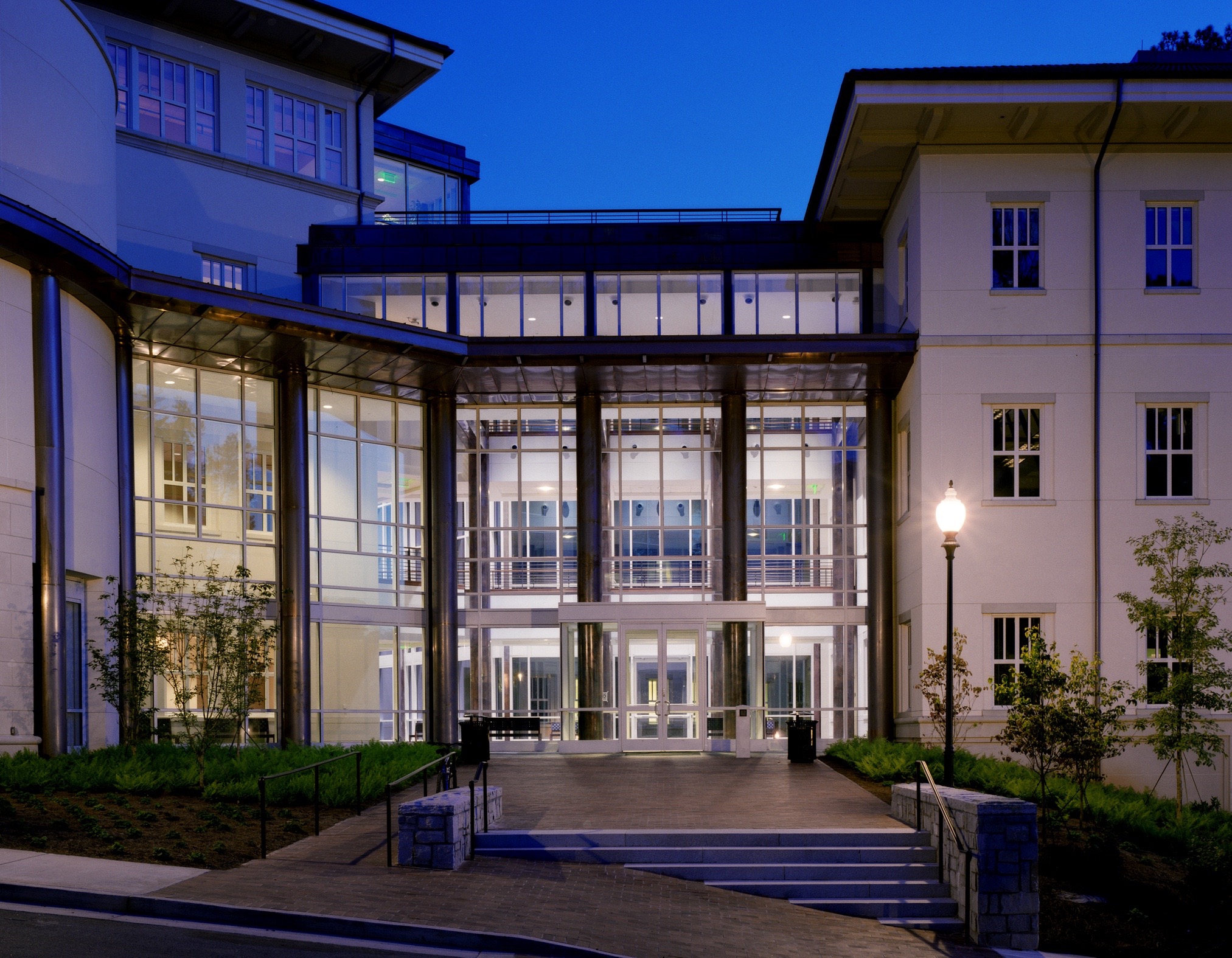
Emory CS is Hiring Faculty for 2024!
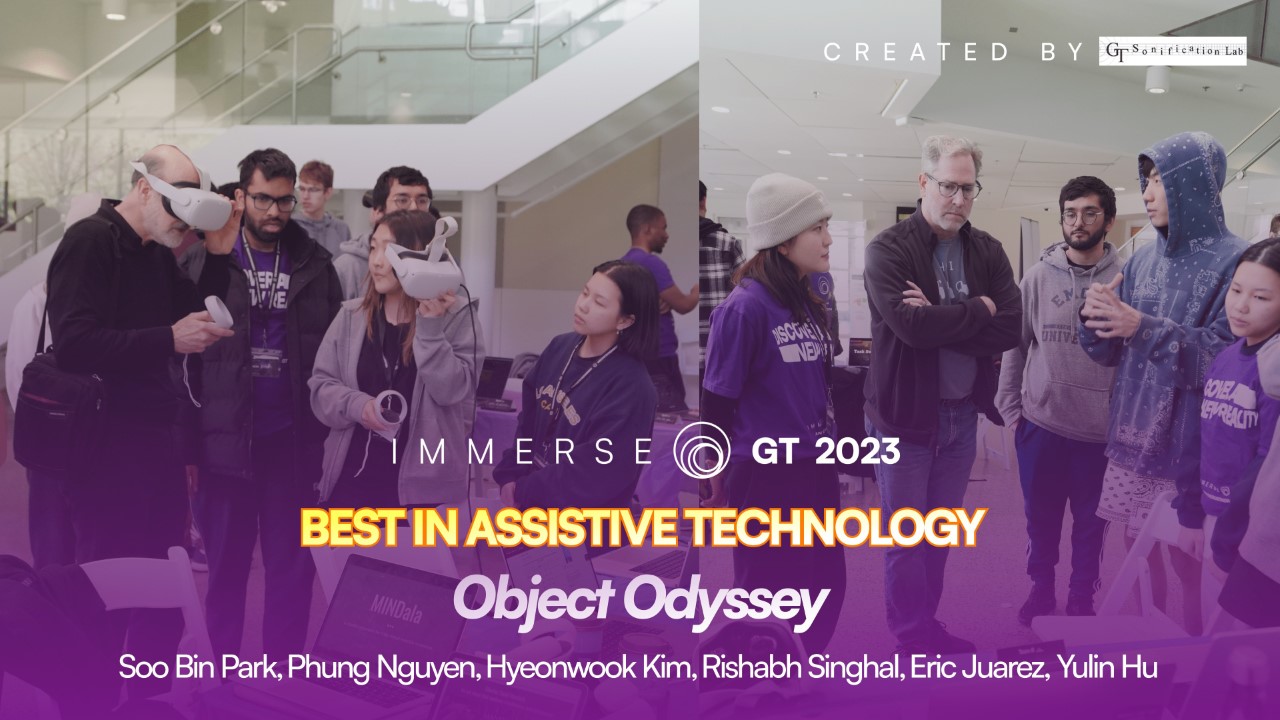
Emory Students Win Awards at ImmerseGT 2023 Hackathon
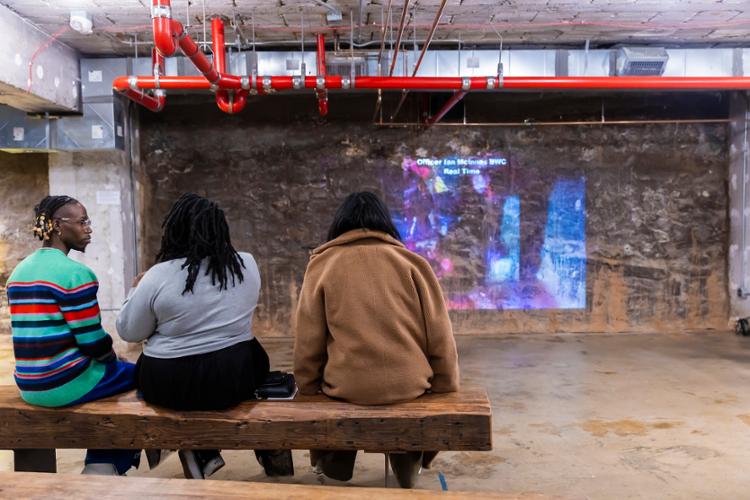
Information Visualization students participated in ASJ
News Archive
CS Undergraduate Awards 2023
Published Date: 2023-05-02
On May 2, we came together to celebrate our sensational seniors! This year’s award winners were presented with their certificates by the faculty members who had nominated them. The winners are as follows:
• Alexandru Rudi - Outstanding CS Student of the Year. Alexandru has taken a position as a software engineer at Jane Street in New York.
• Elijah Chou - Computer Science Chair’s Award for Academic Excellence. Elijah will be staying at Emory as part of the 4+1 program.
• Siyi (Carrie) Gu - Computer Science Award for Academic Achievement. Carrie is off to California to pursue a Masters in CS at Stanford.
• Noah Okado - Computer Science Award for Academic Achievement. Noah is also moving west for a PhD in Computational Neuroscience at Caltech.
• Minxing (Matt) Zhang - Computer Science Award for Academic Achievement. Matt is moving to North Carolina for a PhD at Duke.
• Chen Gong - Computer Science Undergraduate Research Award. Chen has accepted a place at Yale for a Masters in CS.
• Tiantian Li - Computer Science Undergraduate Research Award. Tiantian will embark on a CS PhD here at Emory with Dr. Joyce Ho.
Congratulations to all of our awardees and their CS Faculty Advisors! We are immensely proud of you all and have no doubt that you will go on to do great things!
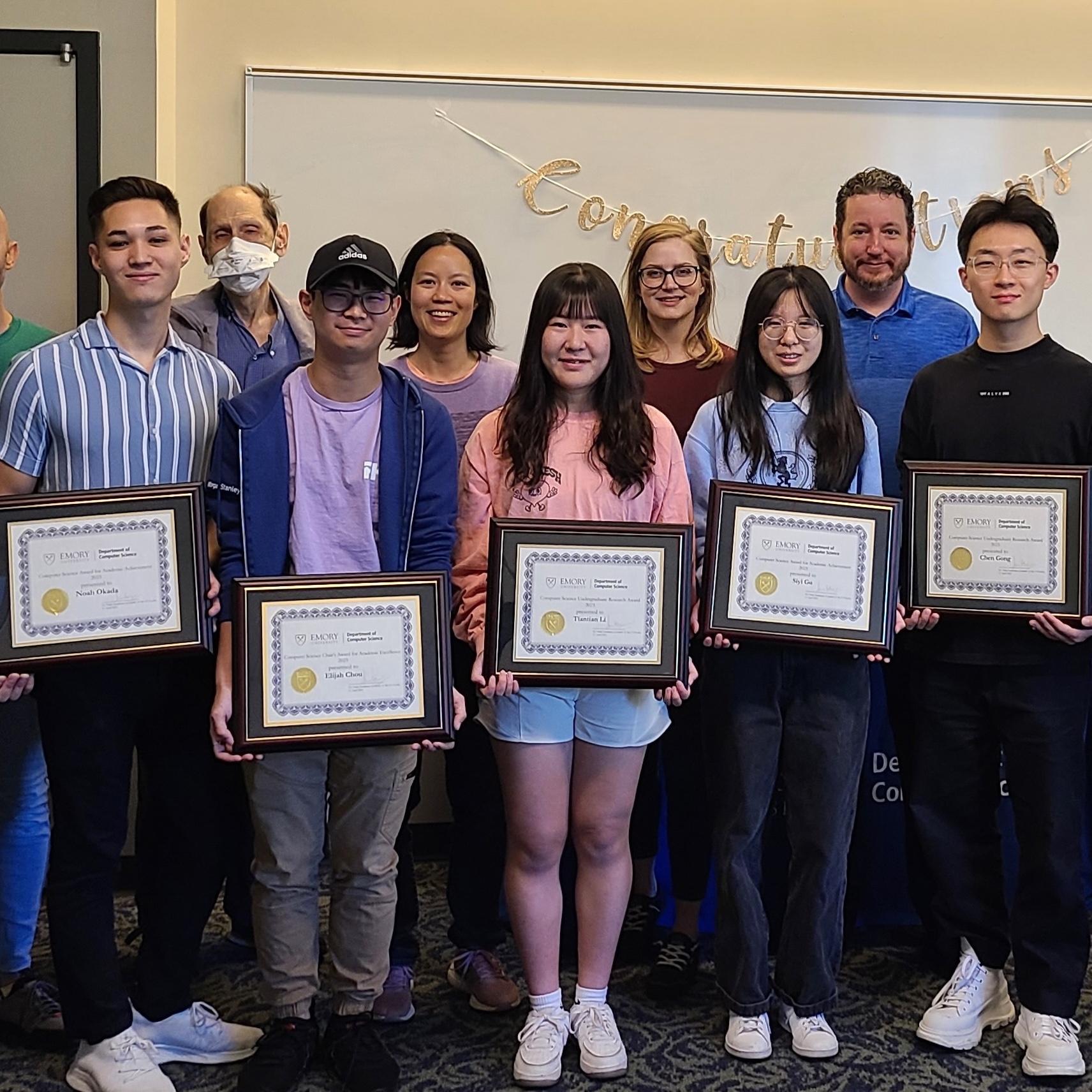
Emory CS Students Bring Home Multiple First Place Awards at ImmerseGT 2023 Hackathon
Published Date: 2023-04-12
Over the weekend, Emory CS students, Yulin Hu, Bella Li, Tye O’Brien, Muzhi (Andy) Liu, Naye Li, Ruby Wu, Yousef Rajeh, and Eric Juarez, participated in ImmerseGT 2023 Hackathon. In total these 8 students were spread across 3 teams, all of whom won first prize in one of the competition categories! Yulin Hu and Eric Juarez were part of the winning team Object Odyssey for the Best in Assistive Technology category. Bella Li, Tye O’Brien, Ruby Wu, Naye Li, and Muzhi (Andy) Liu were part of the winning team MINDala for the Best in Mindfulness category. Yousef Rajeh was part of the winning team SageVR for the Best Use of Omniverse category. Congratulations on your achievements!
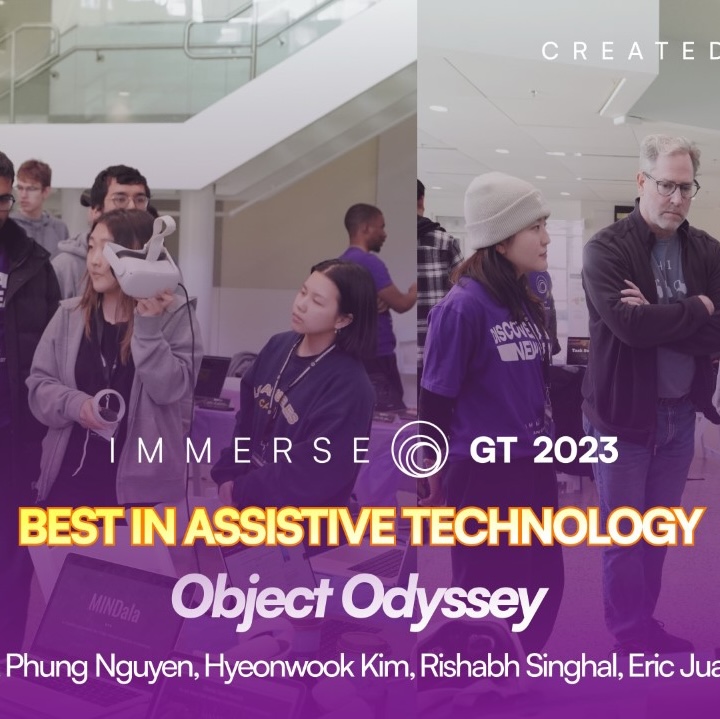
Congratulations to Wenjing Ma, Jiaying Lu and Professor Hao Wu
Published Date: 2023-04-05
Wenjing Ma (CSI PhD'19) has developed a novel computational method with open source software called Cellcano to identify cell types for single cell ATAC-seq data. Cellcano is based on a two-round supervised learning algorithm, and provides significantly improved accuracy, robustness, and computational efficiency compared to existing tools. Cellcano is a joint work led by Wenjing Ma and Professor Hao Wu, in collaboration with another CSI PhD student Jiaying Lu. Cellcano has been accepted by Nature Communications and can be found here. Congratulations!
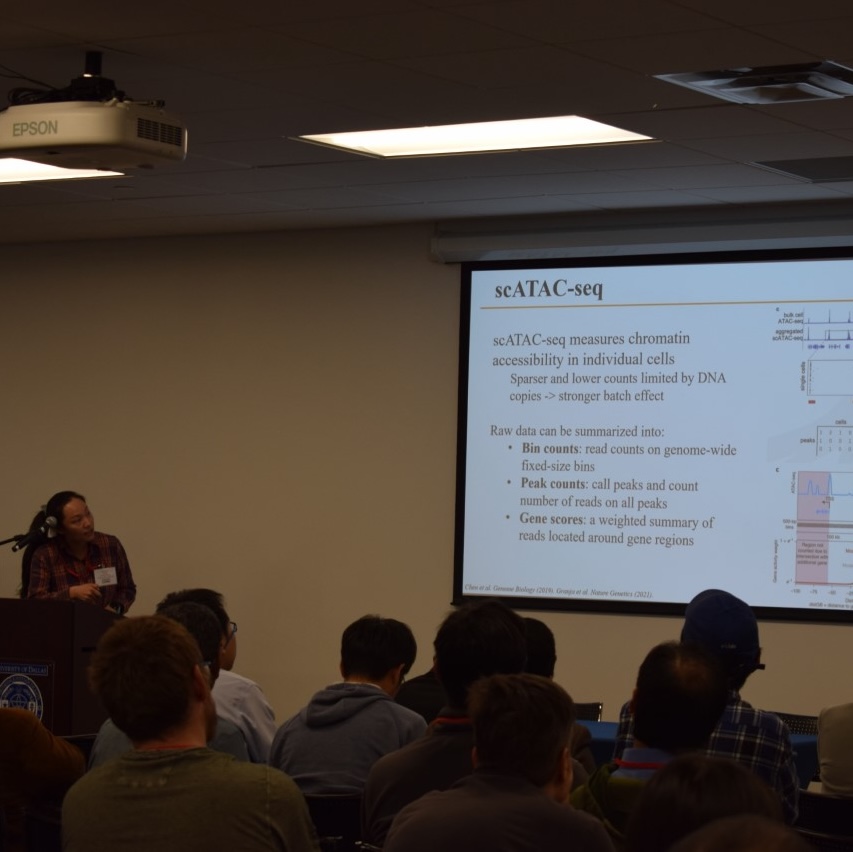
Emory unveils interdisciplinary AI minor open to all undergraduates
Published Date: 2023-03-15
Emory will offer an innovative new minor in artificial intelligence (AI) beginning fall 2023. The program is open to undergraduate students in all disciplines who want a fundamental understanding of what AI is, how it can be used, its intended and unintended consequences and, most important, its interplay with human, societal and ethical issues such as fairness and bias. Original article from the Emory Report: https://news.emory.edu/ebulletin/emory-report/2023/03/15/index.html?utm_source=Emory_Report&utm_medium=email&utm_campaign=Emory_Report_EB_031523
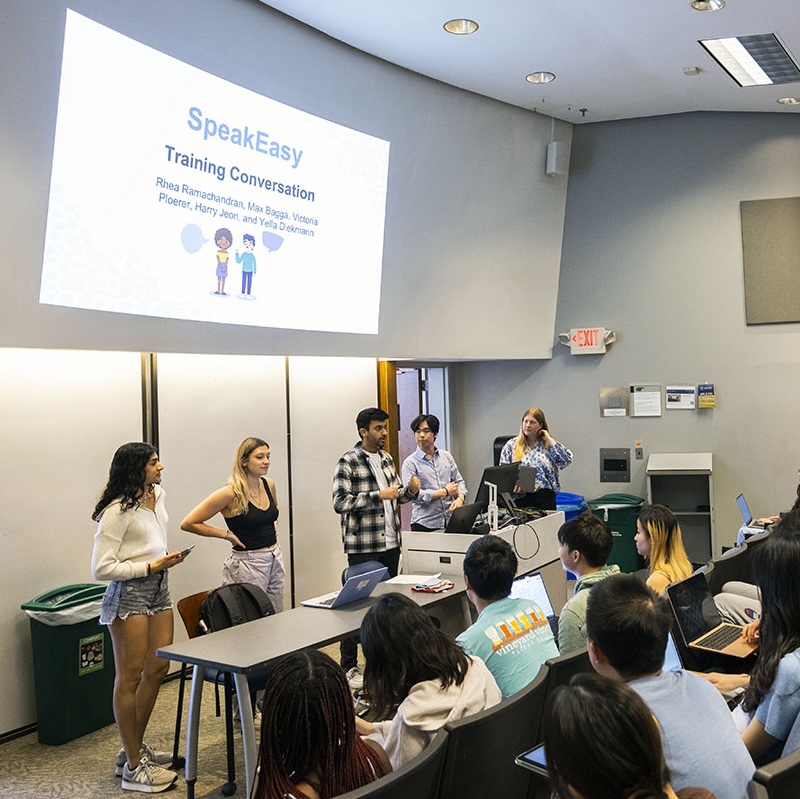
Awards from the ICPC Southeast USA Regional Contest
Published Date: 2023-03-07
Congratulations to our M||E team who won the gold medal for Augusta site and the bronze medal at the International Collegiate Programming Contest: Southeast USA Region. It is the fourth consecutive year that the Emory team has been among the top-3 for Division 1, the most comparative league in this region. The contestants and coaches pictured below are: Alexandru Rudi (Senior), Simon Bian (Junior), Harry He (Junior), Jinho Choi (Coach), and Michelangelo Grigni (Co-Coach). Congratulations to the students who have worked really hard to make this achievement. This team will go to the North American Championship to compete among the top schools in the US in May.
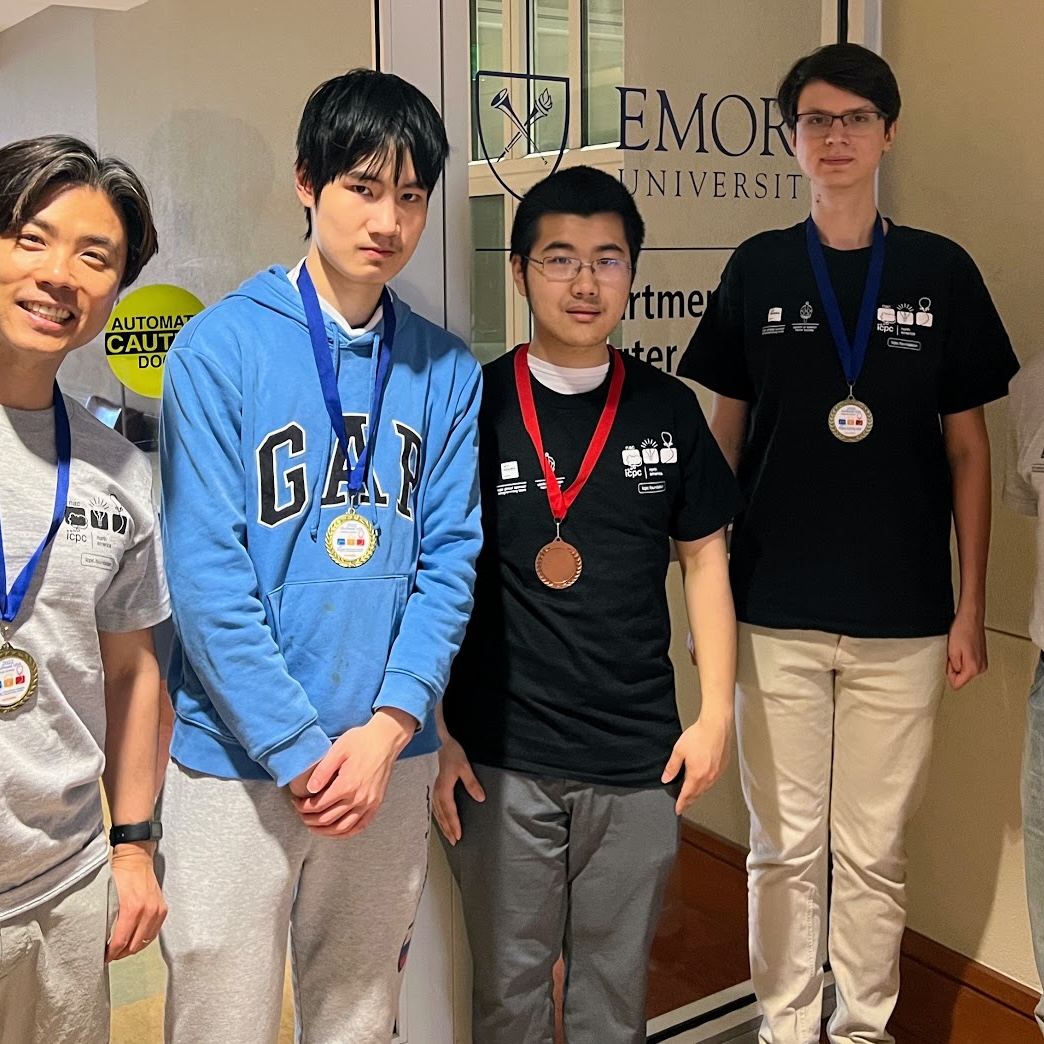
Research Recognition
Published Date: 2023-01-05
Emory undergraduate CS student, Minxing Zhang, was awarded the Best Poster Runner-Up Award for his first authored paper, 'Deep Geometric Neural Networks for Spatial Interpolation' at ACM SIGSPATIAL 2022, a top conference in spatial data science. Zhang is seen here with his advisor, CS Professor Liang Zhao, who is also co-author of the award-winning paper. Congratulations Minxing and Dr. Zhao! For more information on these research projects, please visit https://cs.emory.edu/~lzhao41/index.htm
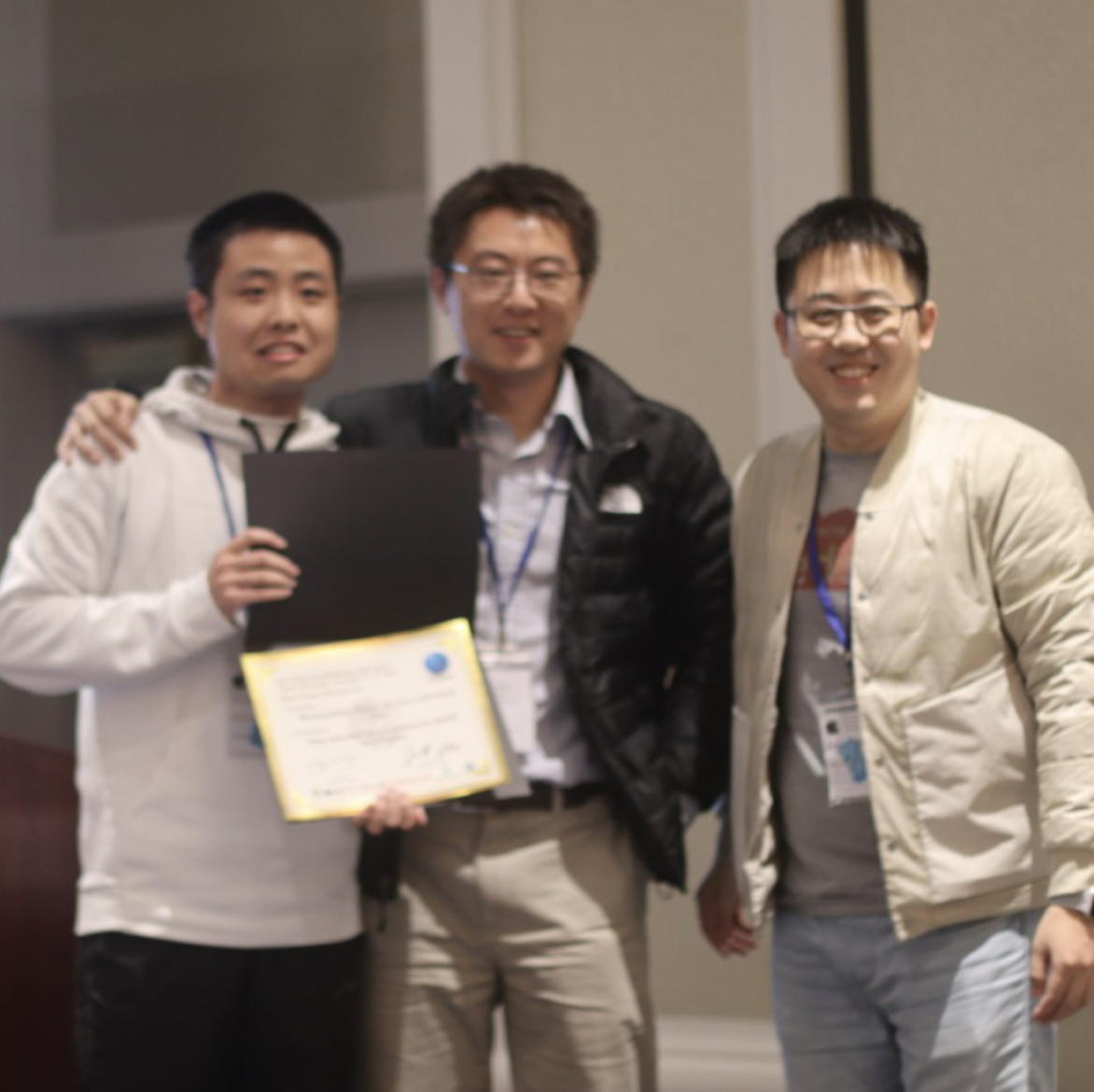
Best Paper Award
Published Date: 2023-01-05
Emory CSI PhD student, Zheng Zhang, won the Best Paper Award for his first authored paper 'Unsupervised Deep Subgraph Anomaly Detection' at the IEEE International Conference on Data Mining (ICDM), a top research conference in data mining. Zhang is seen here with his advisor, CS Professor Liang Zhao, who is also co-author of the award-winning paper. Congratulations Zheng and Dr. Zhao! For more information on these research projects, please visit https://cs.emory.edu/~lzhao41/index.htm
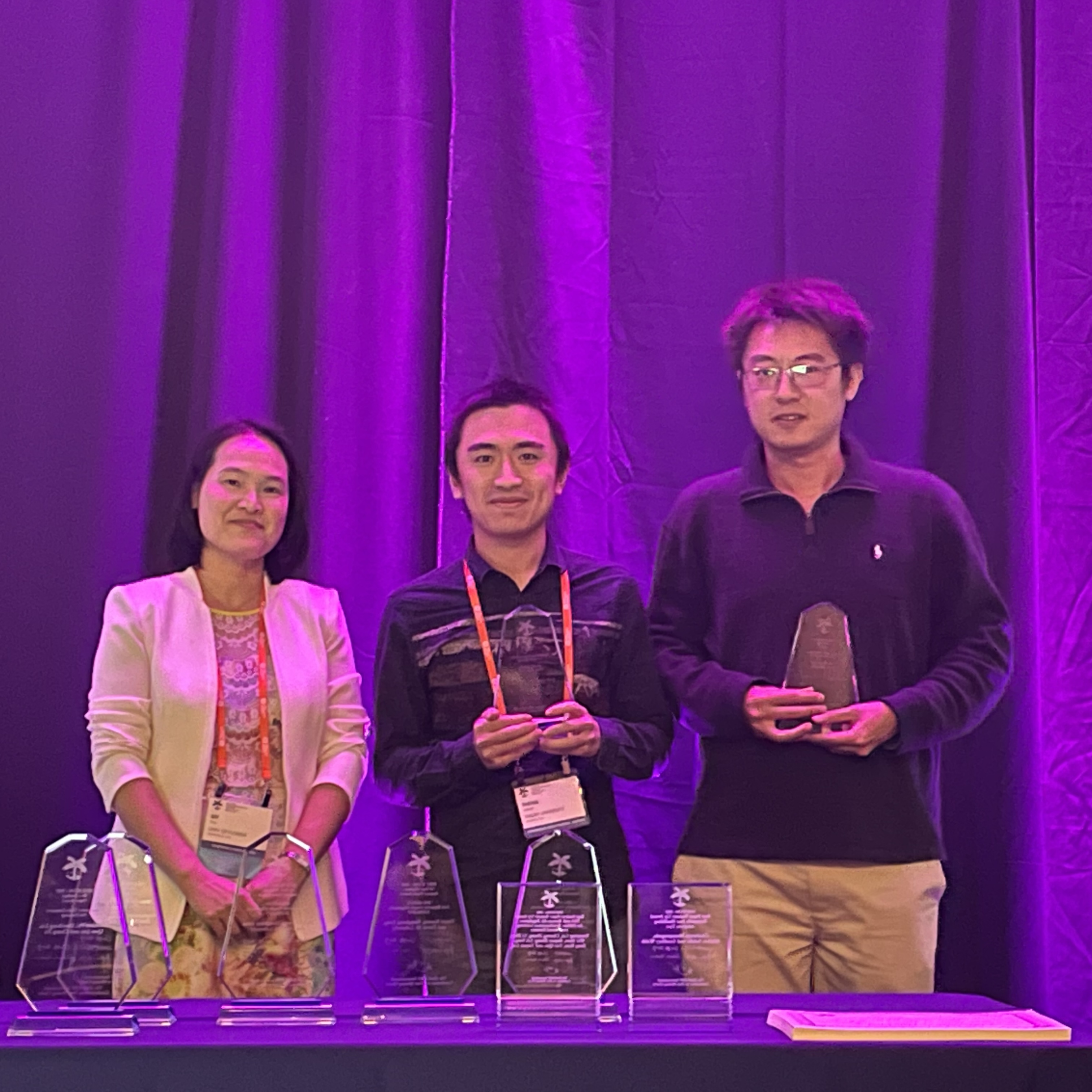